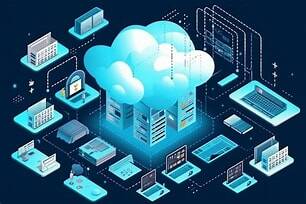
Generative AI in the Cloud: Key Insights and Trends
Generative AI is one of the most transformative technologies today, and its integration with cloud platforms is unlocking new opportunities for businesses. Cloud providers such as AWS, Microsoft Azure, and Google Cloud are leading the charge by embedding AI capabilities directly into their platforms. These integrations make it easier for organizations to build, deploy, and scale AI-driven applications, democratizing access to advanced AI models and tools without needing specialized infrastructure.
1. Key Cloud Providers & Their AI Integrations
- AWS: AWS offers a range of generative AI services under its Amazon SageMaker suite, including tools for natural language processing (NLP), image generation, and even Amazon Bedrock—a service for building AI models using pre-trained foundation models. AWS also offers solutions for AI-powered automation and personalized experiences via services like AWS Deep Learning AMIs.
- Microsoft Azure: Azure integrates AI directly into its cloud ecosystem through Azure AI, including the Azure OpenAI Service, which gives users access to OpenAI’s GPT models, DALL·E for image generation, and Codex for code generation. Microsoft’s investment in AI tools is also reflected in its collaboration with GitHub Copilot, which helps developers write code using AI-assisted features.
- Google Cloud: Google Cloud has focused on making AI accessible with products like Vertex AI for model development and deployment. Additionally, Google integrates advanced NLP and image generation tools such as PaLM (Pathways Language Model), and BigQuery ML, enabling companies to leverage AI for business analytics.
2. Key Aspects of Generative AI in the Cloud
a. Integration of AI with Cloud Services
Cloud platforms are increasingly providing prebuilt AI models and tools that businesses can plug into their existing workflows. These models are trained on vast datasets and offer various capabilities, such as:
- Natural Language Processing (NLP): AI models can analyze and generate human-like text, enabling everything from chatbots to automatic summarization, sentiment analysis, and more.
- Image and Video Generation: Models like DALL·E (OpenAI) and Google DeepMind’s Imagen offer businesses the ability to generate realistic images, videos, and even 3D models based on text inputs. This is especially useful in creative industries like marketing, gaming, and entertainment.
- AI-Powered Automation: Generative AI in the cloud is helping businesses automate processes like customer support (via chatbots), content generation, and even code generation with tools like GitHub Copilot* or AWS CodeWhisperer.
b. Ethical Implications
With generative AI comes significant ethical responsibility. Bias, misuse, and intellectual property concerns are some of the central issues:
- Bias in AI Models: Since these models are trained on vast datasets that may contain biased or inaccurate information, there’s the risk that the AI will perpetuate or amplify these biases. This is particularly concerning in sensitive applications like hiring, credit scoring, or legal analysis.
- Deepfakes and Misinformation: Generative AI models capable of creating realistic videos and images raise concerns about the creation of deepfakes—hyper-realistic falsifications that can mislead or harm individuals, brands, and even political systems.
- Intellectual Property (IP): Who owns the content generated by AI? Should businesses or individuals hold ownership of AI-generated works? These are key questions being explored by lawmakers, IP offices, and tech providers as generative AI becomes mainstream.
c. Scaling AI Workloads
Cloud platforms offer massive infrastructure scalability, which is essential for running generative AI models at scale. Businesses can:
- Elasticity and Cost Efficiency: Use cloud resources only when needed, paying for compute power and storage on a pay-as-you-go model. This is ideal for running resource-intensive AI models without needing to invest in on-premise infrastructure.
- Distributed Computing: Generative AI models, particularly large ones, can require significant computational resources. Cloud platforms support distributed computing and specialized hardware like GPUs and TPUs (Tensor Processing Units) to accelerate AI training and inference.
- Custom AI Models: Providers like AWS, Azure, and Google Cloud allow users to fine-tune or even train custom models on their cloud infrastructure, enabling businesses to adapt the AI to their unique use cases, whether it's content creation, customer service, or product recommendations.
d. New APIs for Custom Model Development
Cloud providers are increasingly offering APIs and frameworks for developing and deploying generative AI models, which significantly lowers the barrier for entry to advanced AI technologies:
- AWS: Amazon Bedrock enables businesses to build AI apps using a set of foundational models from various vendors, including Amazon’s own models and third-party models from providers like Stability AI, Anthropic, and others.
- Microsoft Azure: Azure offers Azure OpenAI Service, allowing businesses to integrate OpenAI’s GPT models into their applications via APIs. Azure also provides tools for custom NLP models, image recognition, and more.
- Google Cloud: Vertex AI simplifies the development of custom machine learning (ML) models by providing APIs for training and deploying models, along with prebuilt tools for automated machine learning (AutoML). Google also offers APIs for text generation, summarization, and translation.
These APIs make it easy to plug AI directly into existing systems without having to be an AI expert, offering a range of use cases, including: - Content Generation: Automatically generating articles, blogs, social media posts, or even scripts for videos.
- AI-Assisted Design: Using tools like DALL·E for creating illustrations or web design.
- Code Generation and Support: Assisting developers in writing or completing code faster with GPT-4, Codex, or other specialized models.
- Customer Interaction: Chatbots powered by GPT models that can handle complex customer queries, including troubleshooting and real-time recommendations.
3. Use Cases and Applications of Generative AI in the Cloud
- Marketing and Content Creation: Generative AI is revolutionizing content creation, enabling businesses to generate blog posts, social media content, advertisements, and product descriptions at scale. For example, GPT models can create high-quality written content, while image generation models can create visuals based on simple text prompts.
- Customer Support**: AI-driven chatbots can engage in natural, human-like conversations to provide customer service, reducing operational costs and improving response times. This is especially effective in industries like e-commerce, tech support, and finance.
- Product and Service Recommendations: AI models can analyze customer data to generate personalized product recommendations. For instance, using generative models to recommend new music, videos, or products based on user behavior.
- Healthcare: Generative AI is helping with data-driven medical research, including drug discovery and disease detection. Cloud platforms allow healthcare professionals to leverage AI models for analyzing patient data, generating medical reports, and improving diagnostic accuracy.
4. Challenges and Future Outlook
- Model Transparency and Trust: One of the key challenges will be building trust in AI models. Users must understand how models work, the data they were trained on, and their decision-making processes.
- Interoperability: Businesses will need to ensure that generative AI models can integrate seamlessly with their existing tech stacks, whether it’s on-premises systems or other cloud platforms.
- Regulation: As generative AI continues to grow, regulatory bodies will likely play an increasing role in ensuring the technology is used responsibly and ethically. Compliance with data privacy laws, AI accountability frameworks, and IP protection will be key.
- Talent and Skill Gap: While cloud providers are making it easier to deploy generative AI models, there remains a shortage of AI and machine learning experts. Businesses will need to invest in upskilling their workforce or partner with specialized providers to harness AI's full potential.
Conclusion
Generative AI in the cloud is a game-changer for businesses looking to leverage cutting-edge AI technologies without the complexity and cost of managing the underlying infrastructure. By providing easy access to powerful models, scalable resources, and developer-friendly tools, cloud platforms are making it easier than ever to integrate AI into everyday business operations. However, as this technology grows, businesses must also navigate the ethical, regulatory, and practical challenges that come with it to fully realize its potential.
Add comment
Comments